Businesses operate AI and machine learning in the supply chain for the management and optimization of supply chain tasks, like monitoring product quality, maintaining inventory levels, and discovering fuel-efficient delivery routes, more effectively than conventional software. Artificial intelligence (AI) refers to applications that mimic human intelligence and carry out complicated tasks. Machine learning (ML) is one of its subfields, involving systems that learn by consuming large amounts of data instead of being given step-by-step directions. In this blog, we’ll learn how AI and machine learning are applied in the supply chain and investigate the various key challenges and future trends in supply chain management.
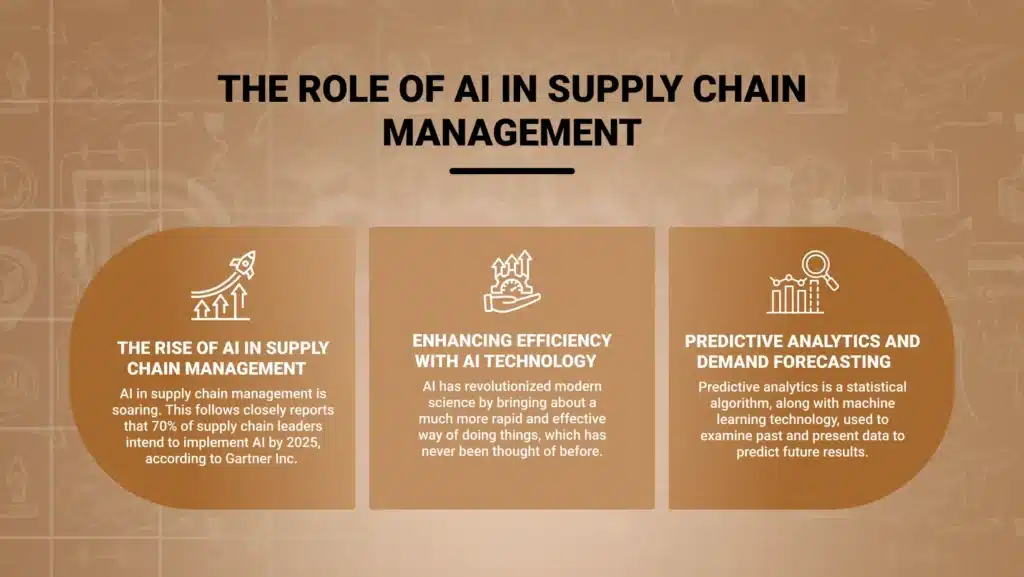
The Role of AI in Supply Chain Management
The main objective of using AI for supply chain and logistics is to increase efficiency and productivity. However, sustainability has increased with the integration of AI into supply chain management, leading all companies to question the potential benefits of digital transformation for their supply chain operations.
Advanced algorithms are utilized to implement AI and ML in the supply chain ecosystem. AI and machine learning in supply chain solutions will improve data quality and provide a completely new perspective on the warehouse and supply chain. Moreover, it can also assist in forecasting requirements and quickly replenishing the ideal inventory levels.
The rise of AI in supply chain management
AI in supply chain management is soaring. This follows closely reports that 70% of supply chain leaders intend to implement AI by 2025, according to Gartner Inc.
Moreover, the growing use of AI in logistics and supply chain management is based on several reasons. First, AI can help businesses gain visibility in the chain. In this sense, businesses can easily identify and solve problems before they precipitate into disturbances. Secondly, AI will enable businesses to run much more efficiently than today. For example, it helps the business optimize inventories, routing, and scheduling. It will help businesses minimize costs and improve efficiency.
The use of AI algorithms can greatly improve customer service by businesses. AI algorithms have the potential to predict demand, track shipments, and solve customer problems. Hence, with the help of AI, this may enable a business to render a better experience for their customers.
Enhancing efficiency with AI technology:
AI has revolutionized modern science by bringing about a much more rapid and effective way of doing things, which has never been thought of before. Its application in industries has reduced human error and caused great productivity and efficiency as well.
AI speeds up the analysis and reporting of data, ensuring accuracy in reducing errors by humans. Since AI works 24/7, its service is constant, quite the contrary of people—therefore, it greatly escalates business efficiency. Arduous processes, such as application deployment, are automated, which further increases efficiency. Generally, AI has metamorphosed businesses into entities that have optimized their performance. Consequently, businesses run with a minimum of errors and effort in the major industries.
Predictive analytics and demand forecasting
Predictive analytics is a statistical algorithm, along with machine learning technology, used to examine past and present data to predict future results. Its pinpoints trends to forecast customer demand and possible business prospects in demand forecasting. Similarly, evidence will be appropriate amounts to be in stock, thereby ensuring there is sufficient stock on hand to supply the demand without simply having idle inventory.
Retailers have quickly embraced predictive analytics in forecasting customer purchases using previous buying patterns, online behaviour, and weather predictions. Furthermore, they improve pricing, promotions, and the management of the supply chain. Manufacturers are using predictive demand forecasting to drive an improved production schedule and procurement of raw materials.
Machine Learning in Supply Chain Optimization
Machine learning applications in the supply chain assist retailers, suppliers, and distributors in implementing crucial transformations required during the pandemic. It really adds the best value to supply chain processes: cost reduction, better risk control, better forecasting, on-time deliveries, and better service. Thus, machine learning has become a major innovation in the field of supply chain management. The following are some great applications that add huge value for the supply chain expert.
Managing a huge number of suppliers, warehouses, and logistics can be quite overwhelming for supply chain management. Moreover, most importantly, machine learning along with AI will be with you to support your full journey of supply chain management. ML algorithms can accurately predict demand, enhance logistics management, streamline paperwork, and automate manual operations. Therefore, you will achieve complete visibility of your supply chain. However, from start to finish, this led to increased efficiency, reduced operational expenses, and improved resilience against disruptions.
AI-Powered Demand Forecasting
Forecasting demand is extremely important in different areas of logistics and supply chain management. Consequently, AI-powered algorithms use past data and market trends in transportation planning to forecast future demand, helping companies improve delivery efficiency by optimizing routes and resources. Similarly, in warehouse space optimization, AI forecasts product demand trends to help manage storage configurations effectively. In supplier collaboration and production planning, AI tools analyze order data and supplier performance metrics to forecast component demand accurately, facilitating streamlined production processes.
Furthermore, in inventory replenishment and reverse logistics forecasting, AI algorithms help minimize stockouts and manage returned products efficiently. Additionally, for cross-border logistics, AI analyzes global trade data to optimize customs clearance and shipping routes.
Importance of accurate demand forecasting in supply chain operations
The connection between demand forecasting and inventory management is closely interconnected since precise demand forecasting enables companies to match their inventory levels with projected demand. Accurate prediction of demand helps businesses prevent negative outcomes like excessive inventory or stock shortages. Excess inventory results in higher storage expenses, devaluation, and discounted sales, leading to financial deficits. However, inventory depletion can lead to dissatisfied customers, lost sales prospects and lost product shortages. Companies can improve the quality of their inventory, reduce carriage costs, and reduce the risk of excess inventory by performing accurate demand forecasts on.
Challenges and Future Trends
AI is revolutionizing supply chain management, making it efficient and agile. Implementing AI in the supply chain still presents some challenges: the obstacles to data quality and integration across disparate systems remain. The second obstacle would be the skills and talent that are needed for proper AI adoption. Modern supply chains come with a sheerness of complexity and scale that is very hard to handle for AI models. Major concerns about data security and privacy arise because sensitive data will pertain to the supply chain.
Moreover, the future of AI in supply chains means moving to predictive and prescriptive insights leading to proactive planning and smart decision-making. Furthermore, this would lead to highly autonomous operations of the supply chain with the least amount of human intervention, where AI will orchestrate activities from inventory, logistics, and fulfilment management. AI can facilitate a dynamic, adaptive supply chain that adapts quickly to disruptions.
Challenges of AI and machine learning in supply chain management
Here are some of the key challenges of artificial intelligence and machine learning in supply chain management. These are:
Data Quality and Integration
AI models are data-hungry entities; hence, they need access to large, good-quality datasets for effective training. Because of silos in data and inconsistent data formats, the integration between the sources of data across the supply chain is still a bad dream.
Skilled Talent Scarcity
A shortage of skilled professionals to develop, implement, and maintain AI and machine learning in the supply chain systems towards the supply chain use case.
Complexity and Scale
A global supply chain can be very complex, with so many entities tied to one another. Developing AI models that can account for this scale is difficult.
Data Security and Privacy
This arises from the very sensitive nature of some data that gets shared when training AI, making data security, data privacy, and information propriety leaks an issue.
Future Trends in AI and machine learning in supply chain management
Here are some key future trends in Artificial intelligence and machine learning in supply chain management. These are:
Predictive Analytics and Prescriptive Insights
Machine learning on historical data can be used to enhance forecasting demand, optimize inventory, and enable predictive maintenance. However, this implies that prescriptive analytics will recommend the best action plans.
Autonomous Supply Chain Operations
AI might finally drive inventory management, logistics routing, warehouse operations, and fulfilment with very marginal human involvement.
AI-driven Supply Chain Networks
AI-enabled dynamic, self-optimizing supply chains will be able to sense and respond to disruptions with the rerouting of inventory and adapting logistics in real time.
Blockchain and AI Integration
The immutability of blockchain and the analytical power of AI could possibly better transparency, traceability, automation, and trust in the supply chain.
Human-Machine Collaboration
While it is true that a few tasks would get automated with the help of AI, people still are the most central part of the decision-making process in supply chains. People should be augmented with AI, and teams should collaborate. Companies that implement AI can secure competitive advantages by enabling their supply chain to operate intelligently, responsively, and efficiently as technologies mature.
Conclusion
Appvin, a leading provider of solutions for supply chain management, plays a vital role in assisting companies in efficiently utilizing analytics. Nevertheless, Appvin Technologies stands out as the best cross platform app development company through its offering of a variety of advanced AI and machine learning in the supply chain tailored particularly for the requirements of supply chain management. This allows businesses to make strategic decisions and achieve operational success by harnessing their data. Appvin’s cutting-edge supply chain analytics platform enables organizations to observe their supply chain operations in real-time, identify inefficiencies, and enhance performance across all aspects. Also, the platform provides information on predicting demand, optimizing inventory, managing transportation logistics, and overseeing suppliers to assist companies in remaining competitive in today’s evolving market.
FAQs
How do artificial intelligence and machine learning enhance the supply chain sector?
Using algorithms, AI provides better quality data, simplifies analysis and gives you a more complete overview of your warehouse and supply chain. Consequently, with the ability to run in a variety of scenarios, AI and ML can provide information about optimal stock levels to meet predicted sales forecasts.
What is the role of AI in supply chain risk management?
The main objective of using AI for supply chain and logistics is to increase efficiency and productivity. Sustainability has increased with the integration of AI into supply chain management, leading all companies to question the potential benefits of digital transformation for their supply chain operations.
What are some examples of AI and analytics in the supply chain?
Some top examples of supply chain data analytics that help optimize operations, support decision-making, and improve operations:
- Forecasting demand
- Warehouse automation
- The method of efficiency
- Supply chain risk management
- Predicted settings
- Supplier personnel management
- Real-time supply chain visibility
What are predictive analytics and how will it impact supply chains?
Machine learning on historical data can be used to enhance forecasting demand, optimize inventory, and enable predictive maintenance. However, this implies that prescriptive analytics will recommend the best action plans.
How might blockchain integrate with AI for supply chains?
The immutability of blockchain and the analytical power of AI could possibly better transparency, traceability, automation, and trust in the supply chain.